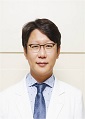
Young Jun Chai
SMG-SNU Boramae Medical Center, South Korea
Title: Ultrasound image analysis using deep learning algorithm for the diagnosis of thyroid nodules
Biography
Biography: Young Jun Chai
Abstract
Background: Fine needle aspiration (FNA) is the procedure of choice for evaluating thyroid nodules and FNA may be required even for nodules with very low suspicion of malignancy if they are larger than 2 cm. However, avoiding unnecessary FNA is important to reduce complications related to FNA and to reduce medical expenses. In this study, we developed an image analysis model using deep learning algorithm and evaluated if the algorithm could predict which nodules would have benign FNA results.
Methodology: Ultrasonographic images of thyroid nodules with FNA cytology or surgical pathologic results were retrospectively collected at Boramae Medical Center, Seoul, Republic of South Korea. The images of the nodules were put into the Inception-V3 network model, pre-trained with ImageNet database for fine-tuning. A total of 1,358 images of the nodules (670 benign, 688 malignant) were used for the algorithm development, and the algorithm was trained to predict a nodule as benign or malignant.
Results: Total 55 nodules (34 benign, 21 malignant) from SMG-SNU Boramae Medical Center, and 100 nodules (50 benign, 50 malignant) from Kuma Hospital, Kobe, Japan were used for internal and external test sets. For internal test set, of the 21 FNA malignant nodules, 20 were predicted as malignant by the algorithm (sensitivity, 95.2%). Of the 22 nodules algorithm called benign, 21 were FNA benign (negative predictive value, 95.5%). For external test set, of the 50 FNA malignant nodules, 47 were predicted as malignant by the algorithm (sensitivity, 94.0%). Of the 31 nodules algorithm called benign, 28 were FNA benign (negative predictive value, 90.3%).
Conclusions: The deep learning algorithm had a high sensitivity and negative predictive value despite an unrealistically high percentage of FNA suspicious for malignancy nodules tested. Using deep learning algorithm may assist clinicians in selecting those nodules that are most likely to be benign and avoid unnecessary FNA.